Synthetic Data Uncovered: Harnessing Artificial Insights for Real-World Solutions
Learn about how synthetic data is reshaping the approach to data science, AI, and machine learning, offering a unique blend of privacy preservation, bias reduction, and innovative solutions across industries.
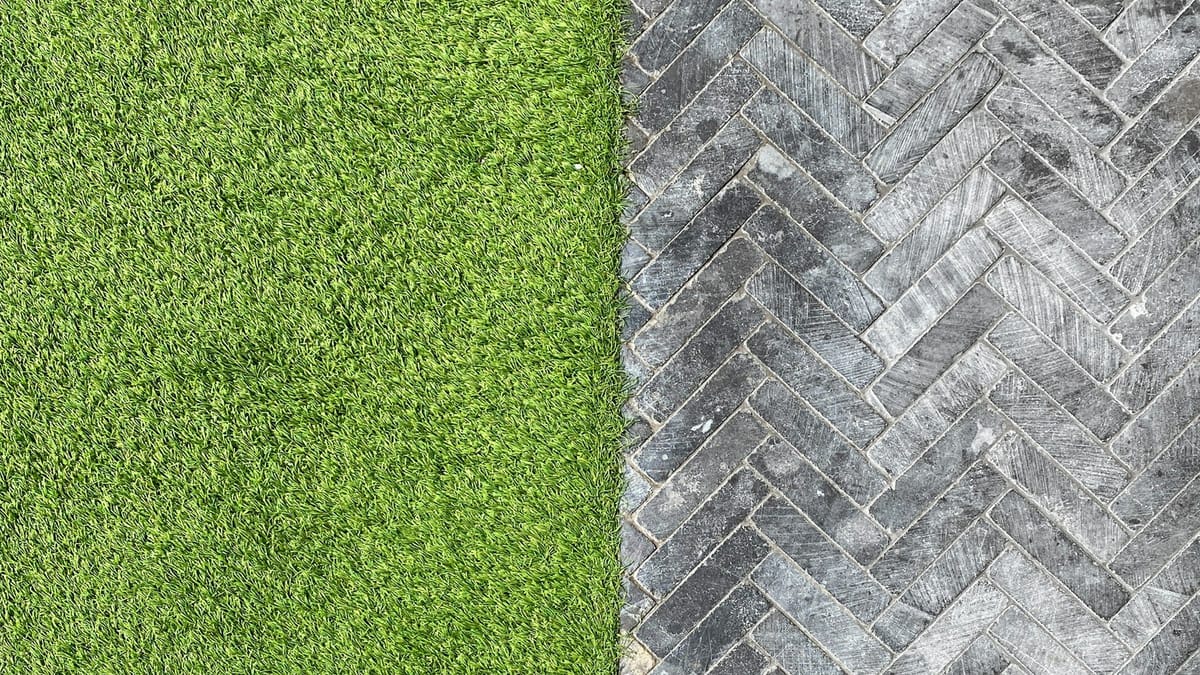
In the digital age, the challenge of harnessing vast amounts of data for AI while maintaining privacy standards is significant. Synthetic data emerges as a critical tool, offering a nuanced approach to this dilemma by advancing technological capabilities and protecting individual privacy.
This article delves into the intricacies of synthetic data, shedding light on its generation process and its substantial impact on both technology and societal norms. Through a focused examination, we explore how synthetic data supports innovation and safeguards confidential information, bridging the gap between data utility and privacy concerns.
As we navigate the complexities of this subject, we aim to provide a comprehensive understanding of synthetic data's role in contemporary and future technological landscapes, emphasizing its importance in ethical AI development and the protection of sensitive data.
Key Takeaways
- Addressing Challenges: Synthetic data, artificially generated, tackles real-world data collection and privacy issues, offering scalable, controllable solutions suitable for AI, machine learning, and privacy preservation.
- Improving Accuracy: It enhances machine learning model accuracy by alleviating data scarcity and bias, with versatile applications across sectors like telecommunications, healthcare, and automotive.
- Quality Assurance: Generation of synthetic data emphasizes accurate replication of original data characteristics, supported by advanced tools and libraries for customized, effective data creation.
Understanding Synthetic Data
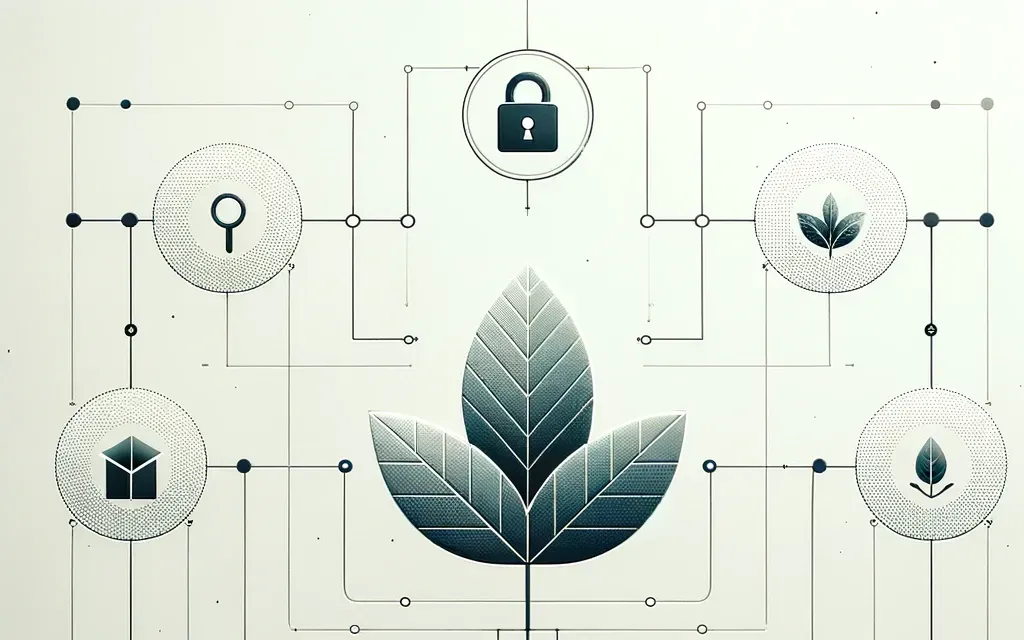
As we delve deeper into the realm of synthetic data, it's important to grasp its foundational concept:
- Artificial Creation: Unlike traditional data derived from real-world events, synthetic data is crafted through artificial means, finding its place across various sectors for tasks like validation and training.
- Privacy and Accessibility Solutions: It addresses significant challenges such as large-scale data acquisition and confidentiality, offering a path to generate data while safeguarding privacy, and making it crucial for data science professionals.
- Replicating Real-World Nuances: The process involves sophisticated AI-driven generative models that replicate real-world data's statistical nuances and correlation patterns with precision, ensuring the synthetic data's relevance and utility.
The benefits of embracing synthetic data are significant:
- Addressing Data Scarcity: It provides a remedy for the paucities encountered in AI research and analytical projects, enhancing the precision in predictive modelling by overcoming the limitations of traditional data sources.
- Future Predominance: By 2030, synthetic data is expected to take precedence in AI and analytics, indicating a transformative shift in data utilization strategies.
- Scalability and On-Demand Generation: The capacity to produce substantial amounts of relevant data as needed bolsters the scalability of data production processes, solidifying the role of synthetic data as an indispensable asset in data science and AI advancements.
Types of Synthetic Data
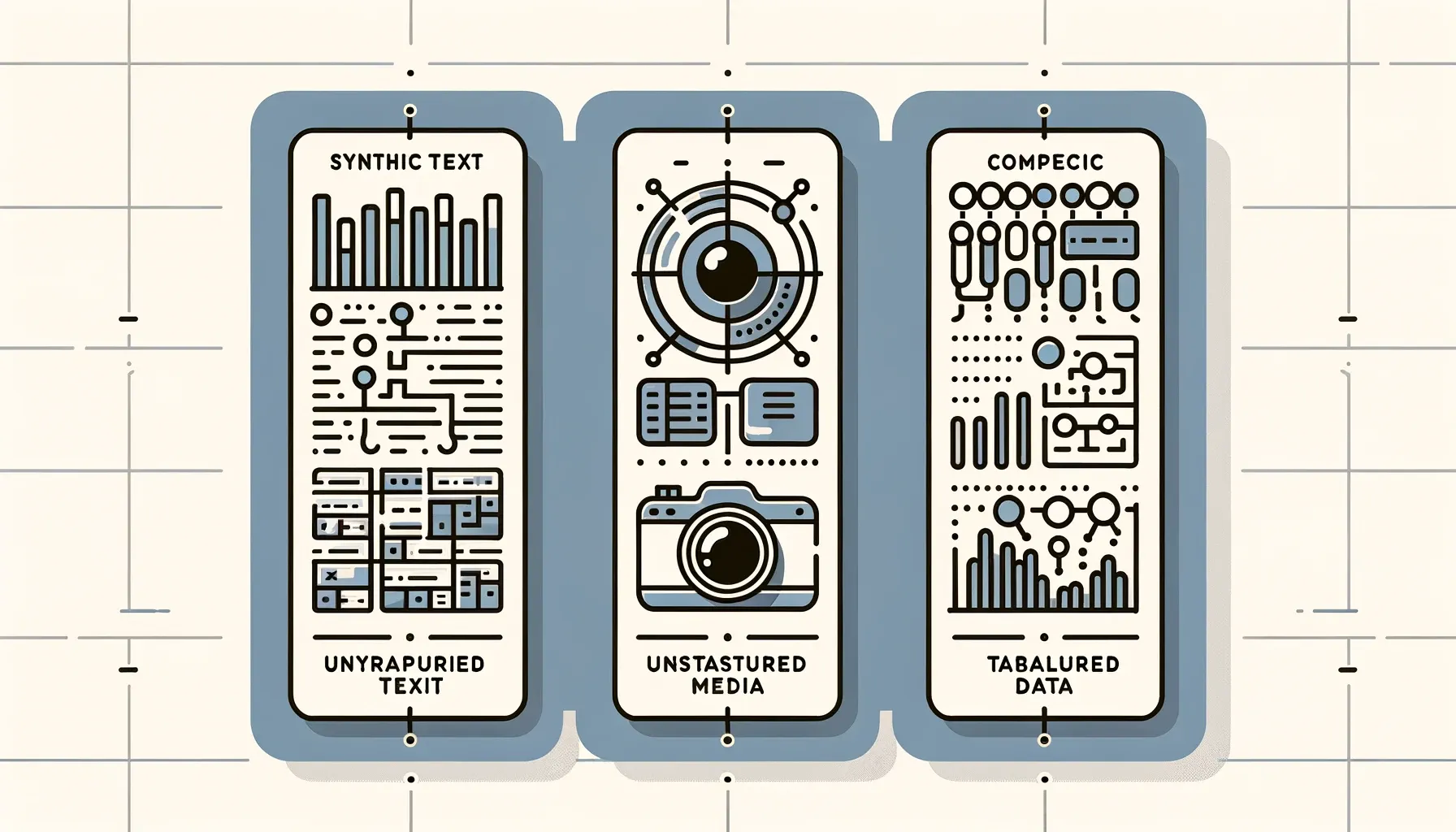
Within the domain of synthetic data, we distinguish two main categories: fully synthetic, created solely through algorithms with no basis in real-world data, and partially synthetic, which merges actual data with synthetic elements to enhance privacy. This categorization serves various purposes:
- Synthetic Text: Tailored for natural language processing, it replicates human linguistic patterns.
- Unstructured Media: Comprises artificial images and sounds for applications in computer vision.
- Tabular Data: Reflects real data structures in a format suitable for analysis and testing.
Crafting these types of synthetic data necessitates a thorough grasp of the original data's characteristics to ensure that synthetic iterations are both useful and privacy-compliant. This strategic creation process enables synthetic data to be versatile and invaluable across different fields, aiding in everything from enhancing machine learning models to ensuring the confidentiality of sensitive information.
Synthetic Data Generation Techniques
To generate synthetic data, a range of sophisticated techniques is applied, each tailored to fulfil specific needs:
- Deep Learning Models: Technologies like generative adversarial networks (GANs) and variational autoencoders (VAEs) are at the forefront, known for their ability to create complex and high-quality synthetic data such as images and sounds. They achieve this by analyzing real datasets and learning underlying patterns.
- Statistical Distribution-Based Methods: This approach involves examining the distributions of real data and creating similar synthetic data by statistically modelling these distributions, ensuring a close resemblance to actual data characteristics.
- Agent-Based Modeling: By constructing models that simulate observed behaviours, this technique produces synthetic datasets that accurately reflect real-world phenomena, adding a layer of behavioural complexity to the generated data.
Each of these techniques contributes uniquely to the synthetic data generation landscape, enhancing its adaptability and versatility.
Applications of Synthetic Data in Machine Learning
Synthetic data plays a pivotal role in machine learning and AI, offering solutions to some of the most pressing challenges in the field:
- Combatting Data Scarcity: It provides an abundant supply of data where real-world data is limited or hard to obtain, ensuring that machine learning models can be trained comprehensively.
- Enhancing Model Accuracy: By adding diversity and volume to training datasets, synthetic data helps improve the predictive performance of AI models.
- Safeguarding Privacy: Acting as a stand-in for sensitive real data, synthetic data allows for model training without compromising individual privacy.
- Mitigating Bias: It offers a means to balance datasets and introduce fairness, helping to reduce inherent biases in machine learning algorithms.
Beyond merely augmenting existing datasets, synthetic data enables the creation of more nuanced and equitable AI models. It supports the inclusion of underrepresented groups in datasets and the application of fairness constraints, contributing significantly to bias reduction. Moreover, its use in simulations for industries such as robotics and autonomous vehicles underscores its value in facilitating secure, controlled training environments.
Training AI Models with Synthetic Data
Training AI models effectively hinges on the availability and diversity of data. Synthetic data emerges as a pivotal resource in this context, offering the flexibility to generate vast amounts of data on demand. This capability not only scales the training and testing of machine learning models but also enriches them by introducing a wider array of scenarios and variations than might be available in real-world datasets.
- On-Demand Generation: The ability to produce large volumes of synthetic data as needed ensures that AI models can be trained under varied conditions, enhancing their robustness and reliability.
- Augmenting Real-World Data: By supplementing actual datasets with synthetic ones, AI models gain exposure to a broader spectrum of data points and scenarios, significantly improving their accuracy and performance.
- Illustrative Success Stories: Leading organizations exemplify the value of synthetic data in AI training. For instance, SyntheticMass provides synthetic patient and population health data for the state of Massachusetts. Amazon HealthLake - provides access to such data for machine learning models.
These instances underline the practical utility and transformative potential of synthetic data in refining AI models across various sectors, paving the way for more nuanced and adaptable AI solutions.
Real-Life Examples of Synthetic Data in AI Projects
Synthetic data is making significant strides beyond theoretical applications, manifesting its value in diverse AI projects across industries. Its practical application is evident in various real-life projects, showcasing its capacity to address complex challenges while safeguarding privacy and enhancing efficiency:
- Autonomous Vehicles: Waymo, an Alphabet subsidiary, leverages synthetic data to refine its self-driving car technologies, generating large-scale synthetic datasets to accelerate development and iteration times.
- Natural Language Processing: Amazon's Alexa AI utilizes synthetic data to advance its NLU systems, especially in new language versions where training data is limited, by creating variations from existing samples.
- Fraud Detection: Financial giants like American Express and J.P. Morgan employ synthetic data to bolster their fraud detection capabilities, enhancing the robustness of their models.
- Healthcare: Anthem collaborates with Google Cloud to develop a synthetic health data platform, enabling the training of AI algorithms while ensuring patient data remains private.
- Clinical Research: Entities like Roche and Charité Lab for Artificial Intelligence in Medicine use synthetic data in clinical research to facilitate data sharing and collaboration, adhering to strict patient data regulations.
These examples underscore synthetic data's transformative impact across sectors, from enhancing the safety and reliability of autonomous vehicles to ensuring privacy in healthcare and financial services, illustrating its broad and practical utility in real-world AI applications.
Privacy and Security Advantages of Synthetic Data
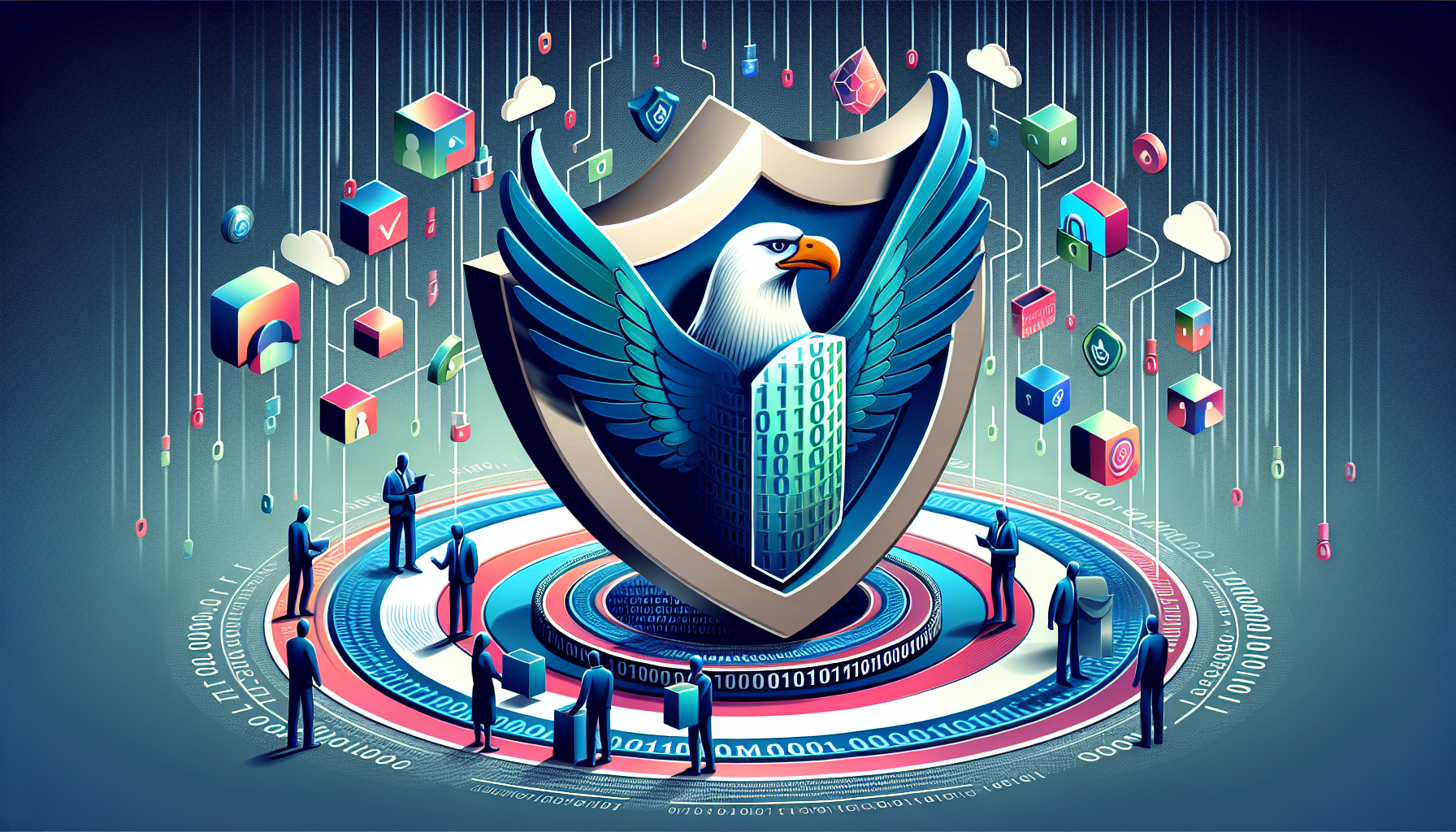
Synthetic data transcends AI and machine learning, crucially impacting software testing and analytics. It offers a cost-effective and efficient alternative to traditional data handling, streamlining the development process.
- Versatile Testing: It's adaptable for various tests like progression and load testing, providing tailored coverage for diverse needs.
- Privacy-Centric: Synthetic data is a secure option for tests, especially where data sensitivity is key, ensuring privacy in non-production settings.
This flexibility makes synthetic data essential for robust, private software testing, boosting system reliability and performance.
Anonymization Techniques Comparison
Synthetic data redefines privacy protection, outpacing traditional anonymization methods like masking or generalization, particularly with complex datasets. These older techniques can compromise data utility and privacy, whereas synthetic data maintains real-world data traits, balancing utility with privacy.
- Enhanced Privacy: Synthetic data ensures data remains useful without risking personal data exposure.
- Rigorous Assessments: Privacy assurance assessments, including metrics like the Nearest Neighbor Distance Ratio (NNDR), verify synthetic data's compliance with privacy regulations, ensuring no sensitive information is discernible.
This approach positions synthetic data as a superior choice for maintaining privacy standards and regulatory compliance, making it pivotal in data-driven industries.
Legal Aspects of Synthetic Data
Synthetic data adeptly meets the demands of privacy and legal compliance, aligning with stringent regulations such as the GDPR. By eschewing personally identifiable information, it operates outside the strictures that apply to real data, embodying the 'data protection by design' approach.
The utility of synthetic data spans various sectors, notable for its legal and compliance advantages:
- Research and Development: In R&D, synthetic data enables innovation and exploration while maintaining strict adherence to intellectual property rights and confidentiality agreements.
- Healthcare: It allows for the analysis of vital health trends and research without compromising patient privacy or violating regulatory constraints.
- Finance: Synthetic data provides a compliant framework for financial models, ensuring transparency and interpretability without sacrificing data integrity.
Endorsements from regulatory entities like the European Data Protection Supervisor and the EU’s Joint Research Center affirm synthetic data's capacity for preserving privacy and minimizing bias, aligning with privacy by design principles and showcasing its critical role in legal and regulatory frameworks.
Synthetic Data for Software Testing and Analytics
Synthetic data's application extends well beyond the realms of AI and machine learning, playing a pivotal role in software testing and analytics. Its generation presents a cost-efficient solution compared to traditional data acquisition and management, saving substantial resources, time, and effort. This efficiency accelerates the development process, as creating synthetic data for testing environments is quicker and less cumbersome than segmenting and anonymizing real-world data.
- Diverse Testing Requirements: Synthetic data can be customized for a variety of testing scenarios, including progression, negative, and load testing. This adaptability ensures comprehensive coverage tailored to specific testing needs.
- Secure Testing Environments: It serves as an ideal alternative to using sensitive real-world data in test scenarios, particularly in settings where confidentiality is paramount. This facilitates secure and effective testing in non-production environments, ensuring data privacy while allowing rigorous evaluation of software and analytical models.
The versatility and customizable nature of synthetic data make it an invaluable asset in ensuring thorough and secure software testing and analytical processes, enhancing overall system reliability and performance.
Removing Human Bias from Data
Synthetic data stands out for its potential to reduce human bias, a critical advantage in the development of equitable and fair AI systems. Through the careful design of synthetic datasets, it's possible to address and mitigate the biases inherent in real-world data. This process ensures the representation of a wide range of demographics, avoiding the pitfalls of skewed data that can perpetuate existing prejudices.
- Diverse Scenario Creation: By simulating a broad spectrum of conditions and scenarios, synthetic data allows AI models to be tested more thoroughly, ensuring their performance is robust and unbiased across different situations.
- Promoting Inclusivity: The deliberate inclusion of varied demographic characteristics in synthetic datasets helps in developing AI technologies that are mindful of diversity, including differences in age, gender, and ethnicity.
This approach leads to the creation of AI models that are not only more accurate but also fairer, reflecting a commitment to inclusivity and equity. By integrating these principles into the foundation of AI training, synthetic data contributes to the advancement of technologies that serve and respect the diversity of the global population.
Cross-Industry Collaboration Opportunities
Synthetic data fosters cross-industry collaborations by offering a platform for secure data sharing, catalyzing innovation without privacy risks. For example, JPMorgan uses synthetic data to efficiently test new ideas with partners, showcasing how such data can streamline collaborative efforts.
- Collaborative Efficiency: Synthetic data speeds up joint ventures, allowing for swift innovation across sectors.
- Privacy Preservation: As a privacy-centric solution, synthetic data enables collaborations in sensitive industries.
Its widespread use underscores synthetic data's role in economic innovation and the creation of new synergies, acting as both a privacy tool and a collaboration catalyst.
Challenges and Limitations of Synthetic Data
Navigating the use of synthetic data comes with its set of challenges, despite its numerous benefits. The creation of synthetic data might not fully capture the complex dynamics of real-world datasets, potentially leading to discrepancies that could impact the effectiveness of models trained on such data.
- Complexity and Randomness: Synthetic data may lack the unpredictability of real-world data, which can influence the performance of AI models.
- Simplification: The process might oversimplify the nuances found in actual data, resulting in a loss of critical details.
- Temporal Accuracy: Emulating the temporal aspects of real data is challenging, with synthetic data sometimes failing to reflect real-time changes.
- Expertise Requirements: Utilizing synthetic data effectively requires specialized knowledge, posing a hurdle for some organizations.
Understanding and addressing these limitations is essential for leveraging synthetic data's full potential in AI and data analytics.
Quality Assurance in Synthetic Data Generation
Quality assurance is paramount in synthetic data generation, aiming to accurately mirror the original data's intricacies. Firms like Mostl AI utilize Model Insight Reports, enabling an in-depth evaluation of synthetic data's fidelity and quality. These reports, armed with precise metrics, offer stakeholders a transparent view of the synthetic datasets' integrity.
- Ensuring Data Integrity: Sophisticated modelling techniques are employed to capture the complex variations of real-world data, maintaining the synthetic data's non-identifiable yet authentic nature.
- Rigorous Output Control: To guarantee the synthetic data's accuracy and consistency, stringent checks are applied, ensuring it aligns closely with the original datasets.
This focus on quality assurance is essential, ensuring that synthetic data remains a reliable and accurate tool for analysis and decision-making across industries.
Tools and Libraries for Generating Synthetic Data
There exists an array of synthetic data generation tools and libraries designed to cater to the varying requirements of this process. Noteworthy options consist of:
- Synthetic Data Vault (SDV): Utilizes both multivariate distribution functions and Generative Adversarial Networks (GANs) to create detailed synthetic datasets.
- Gretel Synthetics: Provides the flexibility for teams to customize the attributes within their synthetic datasets to fit project requirements.
- Plaitpy: Uses YAML files to build complex data configurations, enabling detailed data structuring.
- Mesa: Applies agent-based modelling to generate synthetic data, mimicking real-world processes and interactions.
Such tools offer a breadth of customization possibilities, equipping users with robust capabilities for generating artificial datasets effectively.
For those utilizing Python, there are numerous utilities available that assist in creating synthetic information such as:
- Pydbgen: Offers a wide range of context-sensitive content, supporting multi-language localization for global application development.
- Mimesis: Delivers detailed contextual data with extensive linguistic localization, facilitating international projects.
- Nike’s Timeseries Generator: Specializes in creating realistic time-series data, crucial for temporal analysis.
- Zumolab’s Zpy specializes in simulating image-processing scenarios
These tools and libraries provide robust solutions for generating high-quality synthetic data, catering to a wide array of use cases and ensuring data scientists have the resources needed to simulate realistic datasets effectively.
Summary
Synthetic data is reshaping the approach to data science, AI, and machine learning, offering a unique blend of privacy preservation, bias reduction, and innovative solutions across industries. Its ability to mimic real-world data without compromising individual privacy addresses key challenges in data collection and usage, making it an indispensable tool in the modern technological landscape.
The exploration of synthetic data reveals its versatility in generating accurate, diverse datasets that fuel AI advancements and safeguard sensitive information. From healthcare to automotive industries, synthetic data facilitates ethical AI development and collaborative endeavours, promising a future where data limitations and privacy concerns are significantly mitigated.
As the field of synthetic data evolves, it continues to offer new avenues for overcoming data scarcity, enhancing model accuracy, and fostering cross-industry innovation. Despite facing challenges such as complexity replication and expertise requirements, the ongoing refinement of generation techniques and quality assurance measures ensures that synthetic data remains at the forefront of technological progress. Frequently Asked Questions
Frequently asked questions
What is synthetic data with examples?
A: Artificially generated information, known as synthetic data, serves the purpose of training and testing. This includes computer simulations or algorithmically crafted test collections.
Consider, for instance, the production of unstructured synthetic data in the form of images and videos.
What is the difference between synthetic and artificial data?
A: Artificial data, distinct from both augmented and randomized varieties, is designed to resemble real-world observations for the purpose of training machine learning models when acquiring actual data presents challenges.
What is the difference between original data and synthetic data?
A: Synthetic data generation offers a faster, more flexible, and scalable approach to model data that may not be available in the real world. This is especially important in sectors such as finance where original data might not capture necessary scenarios.
Why is synthetic data useful?
A: Utilizing synthetic data can expedite the advancement, diminish expenses, and enhance the efficacy of machine learning models by providing an abundant amount of top-tier training data for machine learning.
What is a data synthesizer?
A data synthesizer serves as a tool designed to create synthetic data, mirroring the properties of an existing dataset. Its purpose is to enhance cooperation among data scientists and proprietors of sensitive information by offering robust privacy assurances through the implementation of Differential Privacy methods.
Q: How does synthetic data support AI and machine learning?
A: Synthetic data provides diverse, bias-reduced datasets essential for training robust AI models, especially in scenarios where real-world data is scarce or privacy-sensitive, enhancing model accuracy and reliability across various applications.
Q: Can synthetic data enhance privacy in data-intensive fields?
A: Absolutely. Synthetic data plays a crucial role in enhancing privacy by allowing the use of data that resembles real-world datasets without any personal identifiers, making it ideal for sectors like healthcare and finance that are governed by strict privacy regulations.
Q: What challenges come with synthetic data?
A: Despite its benefits, synthetic data generation can face challenges like ensuring the data's complexity and variability accurately reflect real-world conditions, maintaining quality and consistency, and requiring specialized expertise for its creation and application.
Q: Are there specialized tools for creating synthetic data?
A: Yes, there are numerous tools and libraries, such as Synthetic Data Vault (SDV) and Gretel Synthetics, designed for synthetic data generation. These tools provide customizable features to meet specific requirements, aiding in the effective creation of synthetic datasets.
Q: What measures ensure the quality and accuracy of synthetic data?
A: Quality assurance in synthetic data generation involves comprehensive testing against original datasets and employing specific metrics and methodologies to evaluate their fidelity. This process ensures that synthetic data is accurate, reliable, and suitable for its intended use.